
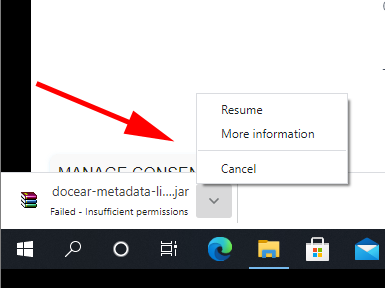
It’s a semi-supervised method of automatic subject labeling of each article at the indexing time itself using a pre-defined classifier hierarchy of major subjects with areas, categories, disciplines, fields and keywords in each area arranged at six levels of hierarchy, which can be utilized to classify research article in any research area and also supports long sequence text search and retrieval. This work proposes a novel subject labeling based academic search engine employing sequence graph-based representation of each article to take advantage of the aforementioned discretized nature of academic search engines. Subject classification is supervised in nature and hence need a taxonomy of subject, fields, and keywords to classify the subject of an article accurately. Search based on research areas or areas of interest needs a proper subject labeling or subject classification of all documents, to retrieve relevant articles belonging to the searched area in a fast and efficient manner. and more preference is usually given to research areas or areas of interest, rather than content-based or other types of queries. Users usually perform article searches in a discretized manner by searching for either paper title, keywords or key-phrases, journal type etc. One of the distinct features of an academic search engine, when compared to other engines besides the category of users surfing for, is the nature of the data they are searching for. This well-defined structure of scientific articles makes it compatible with the academic search engines to represent each article in a graph-based format.
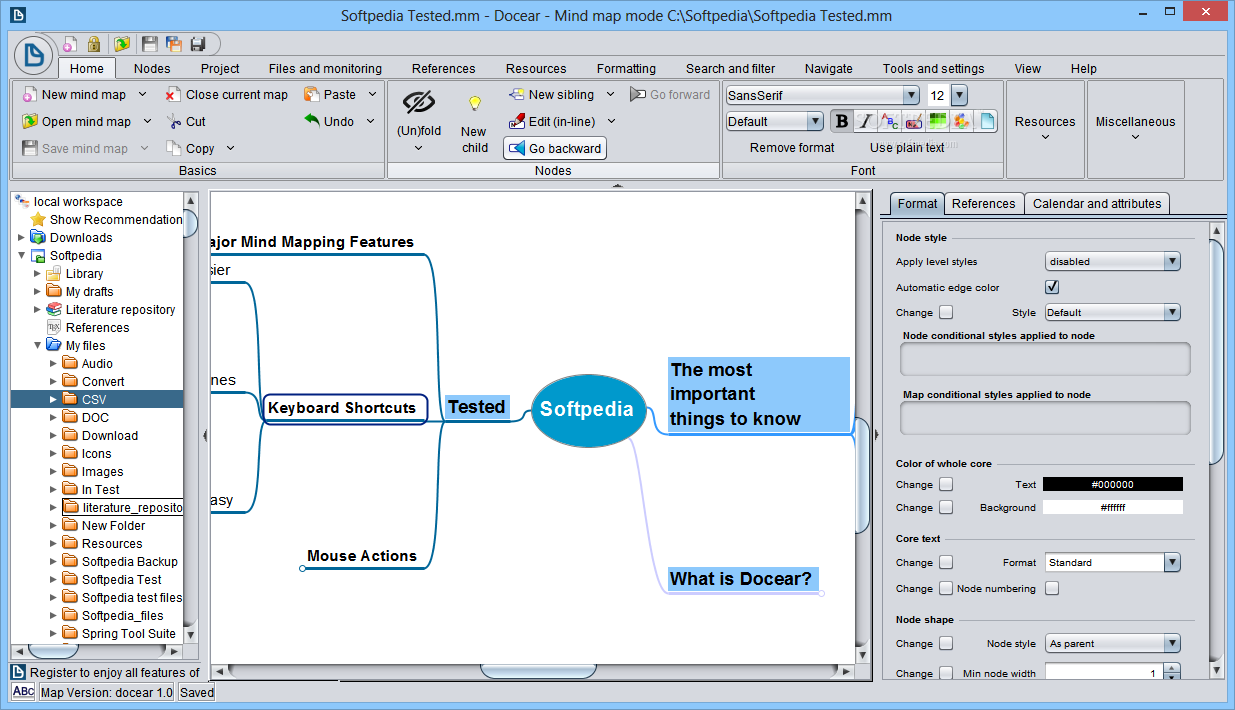
Again, all research papers have a well-defined format when compared to any other text documents with a meaningful title, authors and their affiliations, abstract, list of keywords, document contents including introduction and conclusion, and future scope followed by a list of references.

A major difference is the category of users surfing for, as mainly librarians, academicians or faculties, scientists, researchers, and students are the main users of academic search engines. This is not different in the case of academic search engines too. The existence of search engines solely depends on user satisfaction. The datasets are a unique source of information to enable, for instance, research on collaborative filtering, content-based filtering, and the use of reference management and mind-mapping software. The four datasets contain metadata of 9.4 million academic articles, including 1.8 million articles publicly available on the Web the articles' citation network anonymized information on 8,059 Docear users information about the users' 52,202 mind-maps and personal libraries and details on the 308,146 recommendations that the recommender system delivered. It supports researchers and developers in building their own research paper recommender systems, and is, to the best of our knowledge, the most comprehensive architecture that has been released in this field. for crawling PDFs, generating user models, and calculating content-based recommendations. The architecture comprises of multiple components, e.g. In this paper, we introduce the architecture of the recommender system and four datasets.
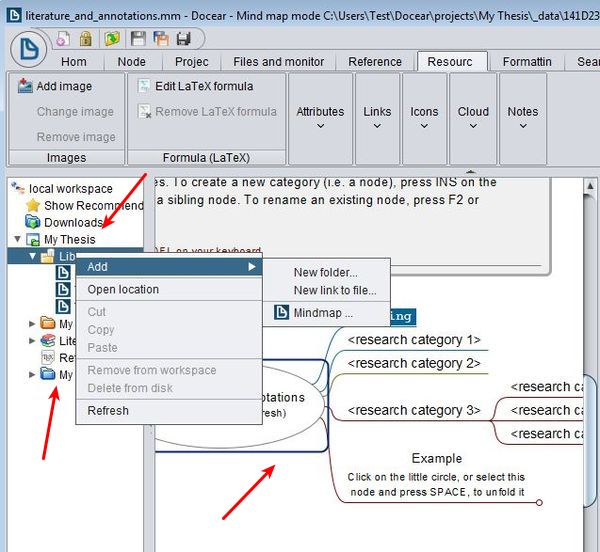
Dino docear software#
In the past few years, we have developed a research paper recommender system for our reference management software Docear.
